Lecture Series
How does language work? Challenges and opportunities in the age of deep learning
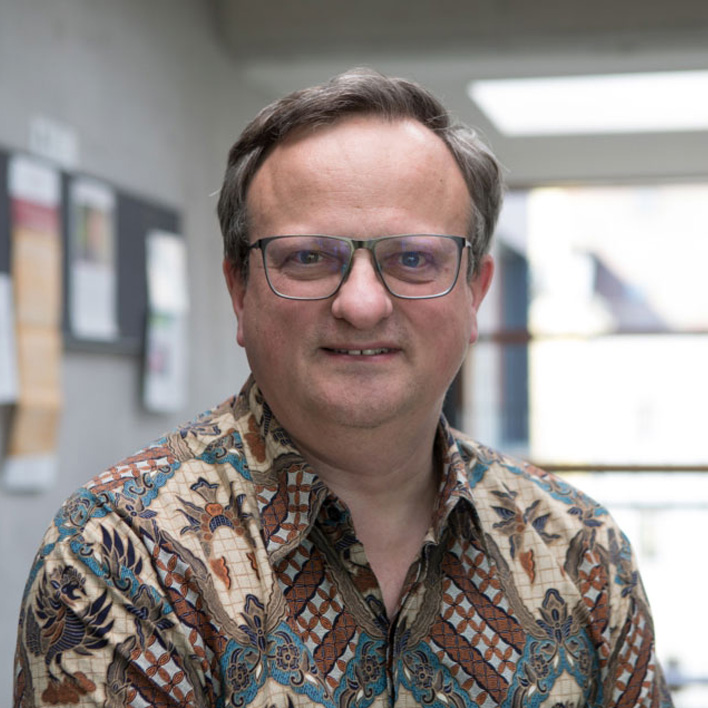
Harald Baayen
Professor of Quantitative Linguistics, Universität Tübingen, Germany
Elected member of the Academia Europaea
Date: 2025/03/20
Time:10:30 - 12:00
Venue:International Conference Room (Jiao 202), Education Building
Organized by National Taiwan Normal University
Co-hosted by the College of Liberal Arts
Abstract
Advances in computer science have deeply influenced the answers that linguists and psychologists have put forward to the question of how language works. The development of formal computer languages in the fifties and sixties led many researchers to conceptualize language as a calculus, a system with elementary units (sounds, morphemes, syllables, words) and rules for combining these atomic units into well-formed sequences. In the eighties, a group of cognitive psychologists started experimenting with artificial neural networks, and argued that it is neural networks that absorb knowledge of language, and not discrete units and combinatorial rules. Many linguists remained unconvinced, and many psychologists felt that neural networks are simply not up to the task. Logic-based and graph-based symbolic approaches were explored instead. Nowadays, artificial networks have made a stunning come-back, and underlie the many large language models that are currently widely used for tasks such as translation and question-answering. These networks are huge, with billions of parameters, and are trained on immense volumes of data. They work extremely well. Deep learning in AI has accomplished what many theorists in linguistics have claimed should be impossible. Are deep learning networks the new model for how language works?
I will argue, on the one hand, that the success of large language models has three important implications for classical, widely accepted, foundational concepts about language and grammar: the idea that the relation between form and meaning is arbitrary, the idea that the sound system of a language is independent of word end sentence structure, and the belief that the language system can be understood independently of general human experience. On the other hand, I will also explain why I do not regard large language models as cognitively valid models. Nevertheless, I do believe that current methods from artificial intelligence have great potential as statistical' tools for uncovering novel layers of systematicity in how language is actually used and learned. To illustrating the above, I will discuss three example problems: How to predict the correct determiner for English, how to predict how tones are pronounced in spontaneous Taiwan Mandarin, and how to predict the time one needs to pronounce Estonian words.
About the speaker
Professor R. Harald Baayen is a distinguished scholar in quantitative linguistics, serving as a professor in the Department of Linguistics at the University of Tübingen, Germany, and an elected member of the Academia Europaea. His research encompasses morphological productivity, language variation, and statistical data analysis, with a particular focus on word structures and their processing in language comprehension and production. As a pioneer in computational and empirical linguistics, Professor Baayen has made groundbreaking contributions to the field through his innovative approaches to statistical modeling.